Current Projects
ASODrugDesignAI: AI-Accelerated Oligonucleotide Drug Design
The clinical use of oligonucleotides requires chemical modifications to increase stability and reduce toxicity; out of the vast spectrum of possible modifications only a very limited amount has been experimentally investigated with respect to impact on thermodynamics. Existing data suggests that alternative modifications can have a sizable effect on safety of drugs. Additionally, the efficiency with which oligonucleotides can pass the cell membrane and be transported to the appropriate location in the cell can be increased with conjugation (binding) to specifically designed small molecules. Also here, prediction of thermodynamics of conjugated oligonucleotides is needed.More...
GenomicSignatures: Alignment-free genome analysis
In an age of global pandemics, studying how viruses and their genomes evolve is of great importance. It has previously been found that genomes of many eukaryotes and prokaryotes have specific preferences for nucleotides, dinucleotides, and codons. Such preferences are characterized by the selective pressure acting on the genomes and are referred to as specific genomic signatures. However, it is not clear to what extent viruses have genomic signatures or to which extent they are shaped by the specific host of the virus or other biological factors.More...
AlgorithmAnimations: Displaying how algorithms compute
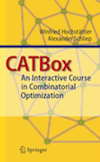
Algorithm Animations are a way of interactively exploring the dynamics of algorithms as they compute solutions. In particular graph algorithms are natural candidates for such animations and indeed there already exists a variety of packages and programs to animate the dynamics when solving problems from graph theory. Still, and somewhat surprisingly, it can be difficult to understand the ideas behind the algorithm from the dynamic display alone. We explore novel animations, technical solutions to the problem of integrating animations into programming exercises and integrating animations with coursework.More...
IDADrugDesign: Intelligent data acquisition for drug design
A problem that occurs in machine learning methods for drug discovery is a need for standardized data. Methods and interest exist for producing new data but due to material and budget constraints it is desirable that each iteration of producing data is as efficient as possible. We investigate Active Learning for models that use the margin in model decisiveness to measure the model uncertainty to guide data acquisition. We demonstrate that the models perform better with Active Learning than with random acquisition of data independent of machine learning model and starting knowledge. We also study the multi-objective optimization problem of combinatorial library design. Here we present a framework that could process the output of gener- ative models for molecular design and give an optimized library design. The results show that the framework successfully optimizes a library based on molecule availability, for which the framework also attempts to identify using retrosynthesis prediction. We conclude that the next step in intelligent data acquisition is to combine the two methods and create a library design model that use the information of previous libraries to guide subsequent designs.More...
Current Projects
Completed Projects
Completed Projects
ComplexDiseases: Analysis of complex disease data
Finding the genetic causes of complex diseases such as Autism and ADHD is complicated by ambiguities and subjectivities in the diagnostic process and the simultaneous involvement of multiple genes and environmental factors. We investigate the application of mixture model based clustering on fused geno- and phenotype data. This joint analysis might yield further insight into the complex interactions between geno- and phenotypes which underlie a specific disease pattern.More...
GenExpTimecourses: Analysis of gene expression time-courses
The molecular processes of life are dynamic over time. Microarray experiments measuring the expression levels of a multitude of genes over time are one way of gaining insight into the dynamic processes. As a first analysis groups of similar expression patterns are routinely identified. We have developed an approach which allows to use prior knowledge, is flexible and very robust to noise. The method is implemented in the software GQL which allows control of the analysis process by use of graphical user interfaces. Currently, we are extending our framework to allow integration of further data related to transcription or protein interactions. Furthermore, we are also investigating methodologies for validating clustering of genes with functional annotation.More...
HTSMethods: Analysis of high-throughput sequencing data
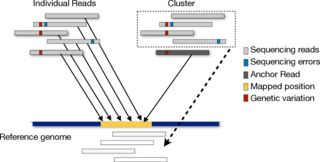
Next generation sequencing experiments produce millions of short reads from target genomes in a cost-efficient manner. Higher throughput brings new challenges such as how to map these short reads efficiently and how to deal with errors introduced by sequencing machines. Currently, we are investigating the problem of read-mapping in an indel-tolerant manner. We are also looking for techniques which will efficiently map reads back to reference genome.More...
ArrayCGH: Analyzing comparative genomic hybridization data
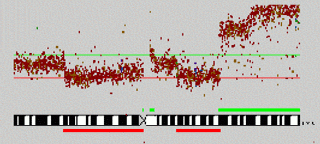
Detecting Chromosomal aberrations from ArrayCGH and gene expression ArrayCGH experimental data Chromosomal aberrations such as deletions or duplications of chromosomal regions are a crucial contributing factor to cancer. The aberrations can be detected by observing the relative hybridization intensities of healthy vs. diseased patients for BAC-clones covering complete genomes. A Hidden Markov Model with a inhomogeneous Markov Chain allows to reflect dependencies between overlapping clones.More...
SCG: Bioinformatics for Single-Cell Genomics
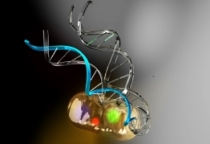
Genome assembly is one of the fundamental problems in Bioinformatics. Assembly can be either reference guided--when we have a reference genome that is similar to the genome we want to assemble--or de novo - when the genome is reconstructed only from reads available from sequencing machines. With sequencing getting cheaper by the day, researchers are interested in assembling genomes of more and more organisms. The main bottleneck here is the lack of reliable de novo assembly tools for Next Generation Sequencing data (the cheaper but shorter reads). We wish to investigate various aspects of the de novo assembly problem such as read filtering and correcting, contig building, scaffolding, etc.More...
CSIMixtures: Context-specific independence mixture modeling for sequence motifs
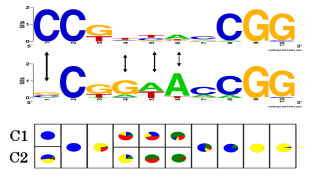
The modeling and analysis of sequence motives is one central task in the elucidation of biological processes such as gene regulation. The choice of model class is crucial to obtain a representation of the motive suitable for the biological application. For instance previous studies showed that for transcription factors which bind to divergent binding sites, mixtures of multiple PWMs increase performance. However, estimating a conventional mixture distribution for each position will in many cases cause overfitting. We avoid this problem by employing a context-specific independence (CSI) framework. In CSI mixtures model complexity is automatically adapted to match the variability found in a given data set.More...
ProteinComplexes: Delineation of protein complexes in yeast
The delineation of protein complexes from protein-protein interaction data is not as trivial as it may seem. We developed a simple probabilistic framework to cluster purifications while preserving the partial order relation among purifications. With a simple graph-based approach motivated by the asymmetric relationship between purifications, we can visualize overlapping components of protein complexes as supported by the experiment.More...
Tiling: Design of Tiling Arrays
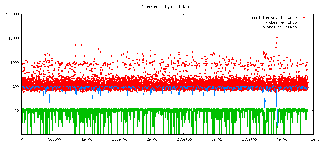
Genomic tiling arrays are universal arrays in the sense that they cover complete genomes or chromosomes uniformly, in contrast to most other types of DNA microarrays for which specific sites of interest such as genes or splice sites are defined a priori. We define the problem of choosing optimal oligonucleotide probes from large candidate sets and provide efficient, linear-time in most instances, algorithms for solving it.More...
MicrorarrayDetection: Detecting biological agents with DNA Micorarray
DNA-Microarrays, well known for measuring gene expression levels, can be used for detecting presence or absence of biological targets (viruses of bacteria) from hybridization patterns of oligonucleotide probes and genomic DNA of agents. Due to sequence similarity of possible targets the use of non-unique oligonucleotides becomes necessary. With use of statistical group testing and phylogenetic information about targets, even the detection of novel targets becomes viable.More...
BayesianHMM: Fast MCMC Sampling for Hidden Markov Models to Determine Copy Number Variations
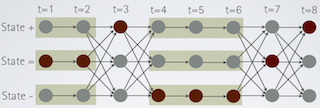
Hidden Markov Models are often used for analyzing Comparative Genomic Hybridization (CGH) data to identify chromosomal aberrations or copy number variations by segmenting observation sequences. For efficiency reasons often parameters of an HMM are estimated with maximum likelihood and a segmentation is obtained with the Viterbi algorithm. This introduces considerable uncertainty in the segmentation, which can be avoided with Bayesian approaches using Markov Chain Monte Carlo (MCMC) sampling. While their advantages have been clearly demonstrated, the likelihood based approaches are preferred in practice for their lower running times. We propose an approximate sampling technique inspired by discrete sequence compression for HMM and kd-trees to leverage spatial relations between data points in typical data sets to speed up the MCMC sampling.More...
DrosophilaDevelopment: Gene regulation during early Drosophila development
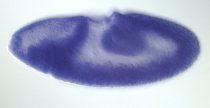
In-Situ Hybridization experiments elucidate the spatial distribution of expressed mRNA in organisms. In particular for Drosophila large amounts of data for several developmental stages are available, complementing the DNA-microarray gene expression experiments. We have developed a image processing pipeline and a framework for joint analysis, which allows to detect co-located co-expressed genes from fused data sets.More...
RemoteHomologues: Identifying clusters of remote homologues
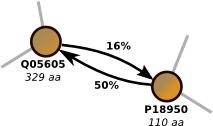
Detecting proteins which share a common ancestor is an important step in understanding protein structure and function. Multi-domain proteins normally cause problems due to spurious similarities they induce; with a simple graph-based approach based on the concept of asymmetric similarity we were able to clearly outperform PSI-Blast.More...
MASCAAT: Meta-Learning for Selection and Combination of Clustering Algorithms Applied to Gene Expression Analysis
Whether to cluster at all, which clustering method to use and how many clusters to choose are pressing questions in bioinformatics. Mostly, decisions are made by users of clustering software based on experience guided by benchmarking or indicators for reliability of solutions or model-fit. However, as clustering algorithms always produce solutions, often inappropriate methods or parameters are used and invalid results produced. Meta-learning refers to the application of machine learning techniques in choosing methods and guiding in setting parameters. We intend to build a computational framework to perform cluster validation and apply meta-learning to the problem of analyzing gene expression time-courses. More information at the Project Page. Joint work funded funded by CAPES (Brazil) and DAAD (Germany) under the program Probral.More...
MouseAtlas: Recognition, analysis and visualization of gene expression patterns in optical tomograms (OPT) of embryonal mice
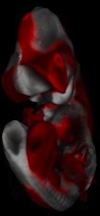
We work in collaboration with Ralf Spörle from the Department of Developmental Genetics, Christian Hege, head of the Visualization Department at the Konrad-Zuse Zentrum (ZIB) and Bernd Fischer, Professor at the University of Lübeck, on the construction of an atlas of gene expression patterns in embryonal mice. The central piece is the construction of a non-linear registration, that maps numerous in-situ tomograms onto an annotated standard model. This mapping yields then an automatical anatomical annotation of high-resolution 3D spatial expression patterns as well as the fusion of all patterns into one standard model. The mapped expression patterns can then be viewed and analyzed together within the standard model. Analysis of the data involves statistical group testing for functional territories.More...
AlgoEngineering: Reduced representations and cache-efficient algorithms and data structures for bioinformatics
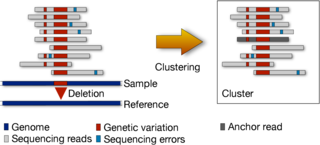
Analyzing large genomic datasets require enormous amount of computational resources in terms of running time, impact on cache, memory and disk space. We are working on finding alternate, reduced representations of these datasets which will enable downstream applications to work much more efficiently. We are also investigating the effect of limited cache on bioinformatics tools, and looking for ways to overcome the difficulties it poses in k-mer counting and read mapping.More...
miRNA: The discriminant power of RNA features for pre-miRNA recognition
Computational discovery of microRNAs (miRNA) is based on pre-determined sets of features from miRNA precursors (pre-miRNA). These feature sets used by current tools for pre-miRNA recognition differ in construction and dimension. Some feature sets are composed of sequence-structure patterns commonly found in pre-miRNAs, while others are a combination of more sophisticated RNA features. Current tools achieve similar predictive performance even though the feature sets used - and their computational cost - differ widely. In this work, we analyze the discriminant power of seven feature sets, which are used in six pre-miRNA prediction tools. The analysis is based on the classification performance achieved with these feature sets for the training algorithms used in these tools. We also evaluate feature discrimination through the F-score and feature importance in the induction of random forests.More...
CellDiff: Understanding transcriptional regulation in cell differentiation
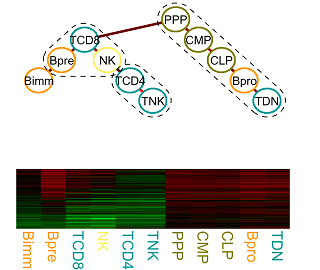
The regulatory processes that govern cell proliferation and differentiation are central to developmental biology. Particularly well studied in this respect is the hematopoietic system. Gene expression data of cells of various distinguishable developmental stages fosters the elucidation of the underlying molecular processes, which change gradually over time and lock cells in certain lineages. We developed a statistical framework for tasks ranging from visualization, querying, and finding clusters of similar genes, to answering detailed questions about the functional roles of individual genes and their similarities and differences.More...